98. Mike Tung - Are knowledge graphs AI’s next big thing?
Towards Data Science - A podcast by The TDS team
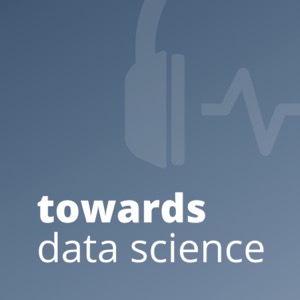
Categorie:
As impressive as they are, language models like GPT-3 and BERT all have the same problem: they’re trained on reams of internet data to imitate human writing. And human writing is often wrong, biased, or both, which means language models are trying to emulate an imperfect target. Language models often babble, or make up answers to questions they don’t understand. And it can make them unreliable sources of truth. Which is why there’s been increased interest in alternative ways to retrieve information from large datasets — approaches that include knowledge graphs. Knowledge graphs encode entities like people, places and objects into nodes, which are then connected to other entities via edges, which specify the nature of the relationship between the two. For example, a knowledge graph might contain a node for Mark Zuckerberg, linked to another node for Facebook, via an edge that indicates that Zuck is Facebook’s CEO. Both of these nodes might in turn be connected to dozens, or even thousands of others, depending on the scale of the graph. Knowledge graphs are an exciting path ahead for AI capabilities, and the world’s largest knowledge graphs are trained by a company called Diffbot, whose CEO Mike Tung joined me for this episode of the podcast to discuss where knowledge graphs can improve on more standard techniques, and why they might be a big part of the future of AI. --- Intro music by: ➞ Artist: Ron Gelinas ➞ Track Title: Daybreak Chill Blend (original mix) ➞ Link to Track: https://youtu.be/d8Y2sKIgFWc --- 0:00 Intro 1:30 The Diffbot dynamic 3:40 Knowledge graphs 7:50 Crawling the internet 17:15 What makes this time special? 24:40 Relation to neural networks 29:30 Failure modes 33:40 Sense of competition 39:00 Knowledge graphs for discovery 45:00 Consensus to find truth 48:15 Wrap-up