64. David Krueger - Managing the incentives of AI
Towards Data Science - A podcast by The TDS team
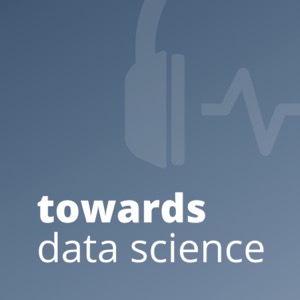
Categorie:
What does a neural network system want to do? That might seem like a straightforward question. You might imagine that the answer is “whatever the loss function says it should do.” But when you dig into it, you quickly find that the answer is much more complicated than that might imply. In order to accomplish their primary goal of optimizing a loss function, algorithms often develop secondary objectives (known as instrumental goals) that are tactically useful for that main goal. For example, a computer vision algorithm designed to tell faces apart might find it beneficial to develop the ability to detect noses with high fidelity. Or in a more extreme case, a very advanced AI might find it useful to monopolize the Earth’s resources in order to accomplish its primary goal — and it’s been suggested that this might actually be the default behavior of powerful AI systems in the future. So, what does an AI want to do? Optimize its loss function — perhaps. But a sufficiently complex system is likely to also manifest instrumental goals. And if we don’t develop a deep understanding of AI incentives, and reliable strategies to manage those incentives, we may be in for an unpleasant surprise when unexpected and highly strategic behavior emerges from systems with simple and desirable primary goals. Which is why it’s a good thing that my guest today, David Krueger, has been working on exactly that problem. David studies deep learning and AI alignment at MILA, and joined me to discuss his thoughts on AI safety, and his work on managing the incentives of AI systems.