Episode 256: Recursive Pollution? Data Feudalism? Gary McGraw On LLM Insecurity
The Security Ledger Podcasts - A podcast by The Security Ledger
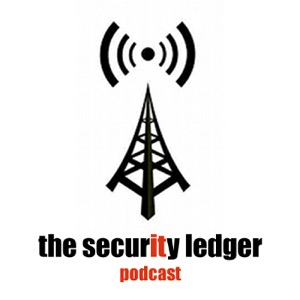
In this episode of The Security Ledger Podcast (#256) Paul speaks with Gary McGraw of the Berryville Institute of Machine Learning (BIML), about that group’s latest report: an Architectural Risk Analysis of Large Language Models. Gary and Paul talk about the many security and integrity risks facing large language model machine learning and artificial intelligence, and how organizations looking to leverage artificial intelligence and LLMs can insulate themselves from those risks. [Video Podcast] | [MP3] | [Transcript] Four years ago, I sat down with Gary McGraw in the Security Ledger studio to talk about a report released by his new project, The Berryville Institute of Machine learning. That report, An Architectural Risk Analysis of Machine Learning Systems, included a top 10 list of machine learning security risks, as well as some security principles to guide the development of machine learning technology. Gary McGraw is the co-founder of the Berryville Institute of Machine Learning The concept of cyber risks linked to machine learning and AI – back then – were mostly hypothetical. Artificial Intelligence was clearly advancing rapidly, but – with the exception of cutting edge industries like high tech and finance – its actual applications in everyday life (and business) were still matters of conjecture. An update on AI risk Four years later, A LOT has changed. With the launch of OpenAI’s ChatGPT-4 large language model (LLM) artificial intelligence in March, 2023, the use- and applications of AI have exploded. Today, there is hardly any industry that isn’t looking hard at how to apply AI and machine learning technology to enhance efficiency, improve output and reduce costs. In the process, the issue of AI and ML risks and vulnerabilities -from “hallucinations” and “deep fakes” to copyright infringement have also moved to the front burner. Back in 2020, BIML’s message was one of cautious optimism: while threats to the integrity of LLMs were real, there were things that the users of LLMs could do to manage those risks. For example, scrutinizing critical LLM components like data set assembly (where the data set that trained the LLM came from); the actual data sets themselves as well as the learning algorithms used and the evaluation criteria that determine whether or not the machine learning system that was built is good enough to release. AI security: tucked away in a black box By controlling for those factors, organizations that wanted to leverage machine learning and AI systems could limit their risks. Fast forward to 2024, however, and all those components are tucked away inside what McGraw and BIML describe as a “black box.” So in 2020 we said. There’s a bunch of things you can do around these four components to make stuff better and to under...