Cathy O'Neil on Pernicious Machine Learning Algorithms and How to Audit Them
The InfoQ Podcast - A podcast by InfoQ
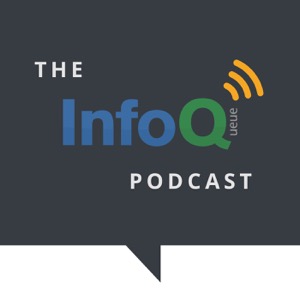
Categorie:
In this week's podcast InfoQ’s editor-in-chief Charles Humble talks to Data Scientist Cathy O’Neil. O'Neil is the author of the blog mathbabe.org. She was the former Director of the Lede Program in Data Practices at Columbia University Graduate School of Journalism, Tow Center and was employed as Data Science Consultant at Johnson Research Labs. O'Neil earned a mathematics Ph.D. from Harvard University. Topics discussed include her book “Weapons of Math Destruction,” predictive policing models, the teacher value added model, approaches to auditing algorithms and whether government regulation of the field is needed. Why listen to this podcast: - There is a class of pernicious big data algorithms that are increasingly controlling society but are not open to scrutiny. - Flawed data can result in an algorithm that is, for instance, racist and sexist. For example, the data used in predictive policing models is racist. But people tend to be overly trusting of algorithms because they are mathematical. - Data scientists have to make ethical decisions even if they don’t acknowledge it. Often problems stem from an abdication of responsibility. - Auditing for algorithms is still a very young field with ongoing academic research exploring approaches. - Government regulation of the industry may well be required. Notes and links can be found on http://bit.ly/2eYVb9q Weapons of math destruction 0m:43s - The central thesis of the book is that whilst not all algorithms are bad, there is a class of pernicious big data algorithms that are increasingly controlling society. 1m:32s - The classes of algorithm that O'Neil is concerned about - the weapons of math destruction - have three characteristics: they are widespread and impact on important decisions like whether someone can go to college or get a job, they are somehow secret so that the people who are being targeted don’t know they are being scored or don’t understand how their score is computed; and the third characteristic is they are destructive - they ruin lives. 2m:51s - These characteristics undermine the original intention of the algorithm, which is often trying to solve big society problems with the help of data. More on this: Quick scan our curated show notes on InfoQ. http://bit.ly/2eYVb9q You can also subscribe to the InfoQ newsletter to receive weekly updates on the hottest topics from professional software development. bit.ly/24x3IVq