Transforming public health with unstructured data and NLP in FDA's Sentinel Initiative
Research in Action - A podcast by Oracle Corporation
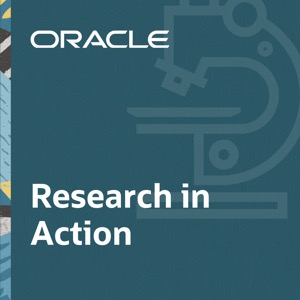
Categorie:
What is the MOSAIC-NLP project around structured and unstructured EHR data? Why is structured data not really enough for drug safety studies? And to what degree is NLP speeding up access to data and research results? We will learn all that and more in this episode of Research in Action with Dr. Darren Toh, Professor at Harvard Medical School and Principal Investigator at Sentinel Operations Center. www.oracle.com/health www.oracle.com/life www.sentinelinitiative.org -------------------------------------------------------- Episode Transcript: 00;00;00;00 - 00;00;26;14 What is the MOSAIC and LP project around structured and unstructured data? Why is structured data not really enough for drug safety studies? And to what degree is NLP speeding up access to data and research results? We'll find all that out and more on this episode of Research in Action. Hello and welcome to Research in Action, brought to you by Oracle Life Sciences. 00;00;26;14 - 00;00;50;14 I'm Mike Stiles. And today our guest is Dr. Darren Toh, professor at Harvard Medical School and principal investigator at Sentinel Operations Center. He's got a lot of expertise in Pharmacoepidemiology as well as comparative effectiveness research and real-world data. So, Darren, really glad to have you with us today. Thank you. My pleasure to be here. Well, tell us how you wound up where you are today. 00;00;50;14 - 00;01;26;22 What what attracted you in the beginning to public health? Good question. So I trained in pharmacy originally, and I got my Masters degree in Pharmaceutical Outcomes Research at a University of Chicago, Illinois, Chicago. And it's where I first learned about a field called Pharmacoepidemiology, which sort of very interesting to me because I like to solve problems with methods and data and pharmacoepidemiology. 00;01;26;22 - 00;02;00;29 It seems to be able to teach me how to do that. So I got into the program at the Harvard School of Public Health, and when I was finishing up, I was deciding between staying in academia and going somewhere and getting a real job. And that's when I found out about an opportunity within my current organization and I've heard great things about this organization. 00;02;00;29 - 00;02;29;26 So I thought I would give it a try. And the timing turned out to be perfect because when I joined, our group was responding to a request for proposal for what is called a mini sentinel pilot, which ultimately became the sentinel system that we have today. So I've been involved in the Sentinel system since the very beginning or before we began. 00;02;29;28 - 00;03;02;25 And for the past 15 years I've been with the system and the program and because I really like its public health mission and I'm also very drawn to the dedication of FDA, our partners and my colleagues to make this a successful program. Well, so now here you are, a principal investigator. What exactly is the Sentinel Operations Center? What's what's the mission there and what part do you specifically play in it? 00;03;02;27 - 00;03;52;26 Sentinel is a pretty unique system because it is a congressionally mandated system. So the Congress passed what is called the FDA Amendments Act in 2007. And within that FDA, the Congress asked FDA to create a new program to complement FDA existing systems to monitor medical product safety and more specifically, the Congress, US FDA, to create a post-market risk identification and analysis system that will be using data from multiple sources that will cover at least 1 million lives to to look at the safety of medical products after they are approved and marketed. 00;03;52;28 - 00;04;33;07 So in response to this congressional mandate, FDA launched what is called a Sentinel initiative in 2008 and in 2009 as I mentioned, FDA issued its request for proposal to launch the Mini Sentinel Pilot program, and the program grew into the sentinel system that we have today. So it's for my involvement. It sort of grew over time. So when I joined, as I mentioned, we were responding to this request for a proposal and we were very lucky to be awarded the contract. 00;04;33;09 - 00;05;04;05 So when it was starting, I serve as a one of the many epidemiologists on the team and I led several studies and I gradually took on more leadership responsibility and became the principal investigator of the Sentinel Operations Center in 2022. So I've been very fortunate to have a team of very professional and very dedicated colleagues within the operations center. 00;05;04;05 - 00;05;27;26 So on a day to day basis, we work with FDA to make sure that we can help them answer the questions they would like to get addressed. And we also work with our partners to make sure that they have the resources that they need to answer the questions for FDA. And most of the time I'm just the cheerleader in chief just to share my colleagues and our collaborators. 00;05;27;28 - 00;06;11;23 Now that's great. And and then specifically, there's the Mosaic NLP project that you're involved with. What is that trying to achieve and what are the collaborations being leveraged to get that done? So Sentinel Systems has always had access to medical claims data and electronic health record data or year data. One of the main goals for the current sentinel system is to incorporate even more data, both structured and unstructured, into the sentinel system and to combine it with advanced analytic methods so that FDA can answer even more regulatory questions. 00;06;11;25 - 00;06;40;09 So the Mosaic and NLP project was one of the projects that FDA funded to accomplish this goal. So the main goal of this project is to demonstrate how billing claims and data from multiple sources when combined with advanced machine learning and natural language processing methods, could be used to extract useful information from unstructured clinical data to perform a more robust drug safety assessment. 00;06;40;11 - 00;07;21;18 When we tried to launch this project, we decided that we would issue our own request for proposal. So there was an open and competitive process, and Oracle, together with their collaborators, were selected to lead this project. So I want to talk in broad or general terms right now about data sharing, the standards and practices around that. It kind of feels silly for anyone to say it's not needed, that we can get a comprehensive view and analysis of diseases and how they're impacting the population without it. 00;07;21;20 - 00;07;46;15 NIH is on board. It updated the DMS policy to promote data sharing. You know, the FDA obviously is leaning into this. So is data sharing now happening and advancing research as expected, or are there still hang ups? So I think we are making good progress. So I think the good news is data are just being accrued at an unprecedented rate. 00;07;46;17 - 00;08;28;21 So there are just so much data now for us to potentially access and analyze. There's always this concern about proper safeguard of individual privacy. And through our work, we also became very appreciative of other considerations, for example, the fishery responsibilities of the delivery systems and payers to protect patient data and make sure that they are used properly. So you mentioned the recent changes, including in data management, ensuring policy, which I think are moving us in the right direction. 00;08;28;26 - 00;08;56;23 But if you look closer at the NIH policy, it makes special considerations for proprietary data. So I would say that we have made some progress, but access to proprietary data remains very challenging. And the FDA, the NIH policy doesn't actually fully resolve that yet. When you think about the people who do make that argument for limited data sharing, they do mostly talk about what you just said about patient privacy. 00;08;56;23 - 00;09;25;20 IT proprietary data. Pharma is especially sensitive to that, I would imagine. So how do we incentivize the reluctant how can we ease their risks and concerns or can we? Yeah, it's a tough question. I think that this require a multi-pronged approach and I can only comment on some aspects of this. So I would say that at least based on our experience, the willingness or ability to share data often depends on the purpose. 00;09;25;23 - 00;09;55;29 That is, why do we need the data? Many data partners participate in Sentinel because of its public health mission, and our consideration is how would the data be used again, Is there proper safeguard of patient privacy and institutional interest? There are other ways to share data. For example, instead of asking the data to come to us, we can send analysis to where the data is. 00;09;56;06 - 00;10;34;22 And that is actually the principle follow by federated system like Sentinel. So we don't pull the data centrally. We send an analysis to the data partners and only get back what we need it. And it's usually in the summary level format. So that actually encourages more data sharing instead of less sharing. I would say that recent advances in some domains, such as tokenization and encryption, might also reduce some concern about a data sharing, a patient privacy concerns in academic settings. 00;10;34;29 - 00;11;24;26 We've been talking a lot about days, for example, for individual who collect the data and the people I propose to offer them authorship or proper acknowledgment if they are willing to share their data. But that is not sufficient in many cases outside of academic settings. If you look at what is happening in the past ten years or so, there are now a lot of what people call data aggregators that are able to bring together data from multiple delivery systems or health plans, and they seem to be able to develop a pretty effective model to convince the data provider to share that data in some way. 00;11;24;29 - 00;11;55;28 And a way to do that could be to help these data providers to manage their data more efficiently or to help them identify individuals who might be eligible for clinical trials. More quickly. So there are some incentives that we could think of to allow people to to share that data more openly but personally, I think that scientific data should be considered public good and hopefully that will become a reality one day. 00;11;56;00 - 00;12;23;21 Yeah, that's really interesting because it sounds like it's both a combination of centralized and decentralized tactics in terms of of data sharing and gathering. Why is it so important to use unstructured data in pharmacoepidemiology studies? And does NLP really make a huge difference in overcoming the limitations and extracting that data? So in the past, I think that that's true. 00;12;23;21 - 00;12;58;07 Now, many pharmaco epidemiologic studies rely on data. They are not collected for research purposes. So we use a lot of medical claims, data that are maintained by payers. We use each our data that are maintained by delivery systems. So this data are not created for research purposes and much of this data, at least for claim, is data stored in structured format using established coding systems like ICD ten. 00;12;58;10 - 00;13;39;06 Coding system and structured data sometimes are not granular enough for a given drug safety study and certain data or set of variables that are required for claims reimbursements or other business purposes might not be collected at all. And people felt that, well, maybe the information that we need could be extracted from unstructured data because as part of clinical care, the physicians or nurse practitioner or the health care provider might include that information in the notes, but use user data also pretty messy, especially that unstructured data. 00;13;39;08 - 00;14;05;25 So instead of going through the unstructured notes manually to extract this information manually, technique by natural language processing could help us do this task much more efficiently so that we can mind a larger model of unstructured data. Well, obviously, when it comes to real world evidence, you're a fan. Tell us what excites you about using it to complement clinical research. 00;14;05;25 - 00;14;42;07 Get us more evidence based insights and help practitioners make better decisions. Yeah, that's a great question. Yes, I'm a fan of so I personally don't quite like the dichotomy between conventional, randomized, controlled trial and real world data studies because they actually sit along a continuum. But is true that conventional randomized trials cannot address all the questions in clinical practice. 00;14;42;09 - 00;15;30;17 So that's where real data and real data studies come in, because real data like we discussed come from clinical practice. So they capture what happens in day to day clinical practice. So if we are thoughtful enough, we will be able to analyze the data properly and generate useful information to fill some of the knowledge gap. The truth is we have been using real data throughout the lifecycle of medical product development for many years now, ranging from understanding the natural history or burden of diseases to using real data as controls for single arm trials, and that we have been doing this before the term real data became popular. 00;15;30;19 - 00;15;57;11 So I see real data to complement what we could do in conventional randomized trials. So real data studies don't replace clinical trials. I see them to be complementary, and real data studies sometimes are the only way for us to get certain evidence. We already talked about Mosaic and LP that project, but I kind of want to go a little deeper with it. 00;15;57;11 - 00;16;42;02 The idea is to tackle the challenges of using link data structured and unstructured at scale. Tell us about a use case for that project and why it was chosen for this project. We actually, Cerner proposed to use the association between Montelukast, which is an asthma drug and neuropsychiatric events as a motivating example. It is also important to note that the project is not designed to answer this particular safety question, because if you look at the label of Montelukast, there's also already a box warning on neuropsychiatric events. 00;16;42;02 - 00;17;18;26 So FDA already has some knowledge about this being a potential adverse event associated with the medication. The reason why or recalls is has proposed this project was because we actually did look at this association in a previous sentinel study that only used structured data, although the study provided provided some very useful information. We also recognized that certain information that we needed was available in such a data, but may be available in unstructured data. 00;17;18;28 - 00;17;42;18 So if we are able to get more data from unstructured data, we might be able to understand this association better. So that's why this motivating example was chosen. Well, this is an Oracle podcast and Oracle is involved in Mosaic, so I think it's fair to ask you about the technology challenges that are involved in what you're trying to do. 00;17;42;19 - 00;18;17;24 What does the technology have to be able to do for you to experience success? So Mosaic in LP is I was at a very ambitious project because it is using an LP to extract multiple variables that are important for the study. That includes the study outcome, which when you look at it, is a composite of multiple clinical outcomes and it's also trying to extract important covariates that could help us reduce the bias associated with real data study. 00;18;17;26 - 00;19;01;24 So I think technology comes in well is powerful in many ways. First, thanks to technology, the project is able to access very large amount of data from millions of patients who seek care in more than 100 healthcare delivery systems across the country. So this was hard to imagine maybe ten or 15 years ago. But now we have access to lots and lots of data at our fingertips because of advances in technology, because of the large amount and the complexity of the data methods side and LP becomes even more important. 00;19;01;26 - 00;19;33;19 And for this project, we are also particularly interested in whether an LP algorithm developed in one year trial system could be applied to another system, which has been a challenge in our field because each year our system is created very differently. So one, an algorithm that works in one system might not work in another. So we are hoping that through advanced methods and technology, we will be able to address this problem. 00;19;33;21 - 00;19;57;15 So without this technology advances, we might not be able to do this study as efficiently as we could all So the task might might not be possible. So where are we going with this? I mean, let's say the project is a success. What will that mean in terms of the FDA's goals and how NLP gets applied in medical therapeutics safety surveillance? 00;19;57;18 - 00;20;38;03 The hope is that Sentinel system can answer even more questions than it can address today. And the way that we are trying to accomplish that is to see whether or how this complex, unstructured data, we combine it with advanced analytic methods can help us answer questions that could not be addressed by structured data alone. I think through this project we also learned a lot about how the challenges associated with analyzing a very large amount of data from multiple sources. 00;20;38;06 - 00;21;11;14 Again, service data is compiled from more than 100 systems, so it is big but also very complex. And in many of our studies we really need that large amount of data just to be able to answer the question because we may be focusing on rare exposures or real come. So you really need to start with very large from our data just to get to maybe the ten patients that are taking a medication. 00;21;11;17 - 00;21;44;15 And what you learn with Mosaic, can that get applied to addressing other public health issues like disparate ease and asthma diagnosis and treatment, especially when you think about diverse groups? Yeah, that's a great question. So is the project is not designed to address these important questions, but if we are able to better understand the completeness of social drivers of health in these data sources, then we will be able to leverage this data to answer these questions in the future. 00;21;44;18 - 00;22;04;26 I think about how a project like this gets a evaluated at various steps along the way. I guess that's my question. How I mean, what what methods are used to ensure the validity of real world evidence? So the good news is in the past few decades we have been using real data, even though we might not be using the term. 00;22;04;28 - 00;22;36;22 So there's been a lot of progress in the field to improve the validity of Real-World Data studies. So we now have a pretty good framework to identify fit for purpose data, and we also have very good understanding of appropriate design and analytic methods. So to target trial emulation and propensity score methods. So this project and many other projects in Sentinel are following this principle. 00;22;36;24 - 00;23;14;03 And one thing to also note that this project is also following the overall sentinel principle in transparency. So everything we do will be in the public domain to allow people to reproduce, so replicate the analysis. So the protocol is available in public domain, and when we are done with the study, everything will be made publicly available. So that's one way to make sure that the the work at least is reproducible or replicable. 00;23;14;05 - 00;23;43;00 And through that process, we hope to be able to improve the validity of this study. And what about comparisons? How do you compare the results from different data sources like claims data, structured data? You know, I extracted unstructured data, all of that. How was that done, the comparisons? So if you're talking about the Mosaic and LP study, so we have a pretty structured approach to address that question. 00;23;43;02 - 00;24;13;14 So we are using this proven principle of changing one thing and keeping everything else fixed to see what happens. So the project will start by using only claims data to replicate the previously done Sentinel study. And then we are going to add on such data to see whether the results are different. And then we add on an LP extract that unstructured data one at a time to see whether the results change. 00;24;13;21 - 00;24;40;24 So by fixing everything else to be constant and changing one thing, we'll be able to assess the added value of each how data, both structure and structure. And that's how we are going to do it within the Mosaic and LP study. And then what about scalability? How would you make sure the NLP models that you develop are scalable and transportable across all these different health systems of which there are many? 00;24;40;27 - 00;25;10;10 Yeah. The question again is about transport ability. So one thing that is unique about this study, as we briefly discussed earlier, was that the the survey yesterday to actually come from multiple healthcare systems. So the end up models that we are developing will be trained in tune on a sample of patients from this system and not from a single hospital network. 00;25;10;10 - 00;25;42;18 So at the development phase, we are already taking into account the potential diversity of different delivery system. And as part of this project, we also include another delivery system to apply and test the method as part of the transport ability assessment. So we are doing that to make sure that the LPI models that we are developing for this project will be useful for other system as well. 00;25;42;20 - 00;26;12;29 Unknown There is a larger question about computational resources, so that will be the issue that would still need to be addressed because a train and tuning this and NLP models within such a huge amount of data requires a lot of computing resources. So that is something that we could only partially address in our study. But if we want to apply or do the same thing in our system, that would be something to consider. 00;26;13;02 - 00;26;43;13 We talked a little bit about the collaboration with your tech partner, but these things usually have so many stakeholders and disciplines and silos. Tell us first why collaboration is a good thing and unavoidable anyway, and then what the challenges of collaboration are. Maybe some tips on how to best make them work. The problems that we face, at least many of the problems that I face quite complex and they require expertise from multiple domains. 00;26;43;13 - 00;27;18;19 So that calls for collaboration from multiple stakeholders. And we always have our blind spots. So we only see things in a certain way and we always miss things. So that's why I think collaboration is important. But it's really hard sometimes because we all have our priorities and perspectives and sometimes they don't align. And I also learned throughout the years that we don't communicate enough and we may also not have time to communicate or we may be under pressure to deliver. 00;27;18;21 - 00;27;47;21 So all of that sort of contribute to the challenges of collaborating effectively, especially when you collaborate across disciplines, because we might be using different languages to mean the same thing or use the same term to describe different things. So even though we can all speak the same language less English, we might not be talking about the same thing and not communicate at all. 00;27;47;21 - 00;28;17;25 Because because we are using different joggers and terminology. So that has been tough. But I think we are getting better. And so I think that it is for us within the center of operation center, we try to communicate honestly and respectfully and we try to understand different perspectives and we try to find common ground. And but I think ultimately what brings us together is that we have a shared common goal. 00;28;17;27 - 00;28;44;17 A lot of the work that we do. So for music and NLP, we are all trying to answer the same question, which is that how do we use unstructured data and advanced analytic methods to answer safety question? So once we apply on this common goal, things become easier because we start to understand each other better or be able to communicate more effectively. 00;28;44;19 - 00;29;19;16 Just out of curiosity, what are the different stakeholders involved in Mosaic? Who falls on the roster? we have people from different disciplines, so we have experts in natural language processing and artificial intelligence. We have epidemiologists, both statisticians, clinicians, we experts in psychiatric conditions and respiratory disease. We have data scientists, we have engineers, we have project managers. So it's a very big group of individuals with different expertise in this project. 00;29;19;18 - 00;29;46;14 Well, you probably noticed Oracle's really thrown itself into and committed huge resources to health and life sciences. Things got really exciting with the acquisition of Cerner and Cerner and Visa. What's Oracle doing right and what do you think it should be doing to make itself even more valuable in health and life sciences? Well, this is a great but very difficult question, so I cannot comment too much what Oracle is doing or will be doing. 00;29;46;17 - 00;30;23;06 But I can say more generally that there have been a number of technology companies that have tried to foray into health or life sciences. I would say with mixed results. And one reason is that our health care system remains highly fragmented and complex, so it takes a lot of energy to break the status quo. So you probably know that we were one of the last countries in the world to transition from ICD nine to ICD ten coding system, and we are soon going to move into the ICD 11 system. 00;30;23;06 - 00;31;00;05 So I'll be interested to see whether the US is ready for that. And that again, is maybe a reflection of just how complex and fragmented our system is and disruptive innovation and I think are great, but they may or may not translate into successes when they applied to health care. That is not to say tempesta mistake. I'm actually pretty optimistic that the perspectives and solutions and ideas brought by technology companies could help us solve a lot of problems that we have today. 00;31;00;07 - 00;31;31;26 But I think that it will be good to engage people who will be struggling with these issues early on and to work together with them to develop solutions that are not just good on paper, but also feasible in practice. So at least in my very limited experience, we have seen some very cool technology that ended up not being useful for health care just because it's very hard to change what people have been doing. 00;31;31;28 - 00;31;56;09 So again, disruptive innovations are good, but sometimes it's just very hard to adopt, at least not quickly enough for for us to see meaningful changes. Yeah, that's really fascinating. It's, you know, it is disruptive innovation, but it's not always applicable to the to the goals you're pursuing. But it does feel like technology where that's concerned, the future is coming at us faster and faster. 00;31;56;11 - 00;32;32;21 So what are the technologies that are most interesting to you? Is it A.I. or what big advances in public health do you see coming? Maybe sooner than we thought. Yeah. Yeah. You know, I feel like you said some of this came too fast. Like, I wish I. And closer to retirement, I don't worry about this. But so even though I say disruptive innovation sometime might not work in health care, but I will say generative A.I. seems to be a recent exception. 00;32;32;24 - 00;33;10;14 So I would say that generative is definitely on the list of things that surprised me in a very nice way. I will also say that the continue fast accrual of better real data is also something that excites me and the continue recognition or increased recognition of the potential real data of. It's also something that I think is good to have for things that came sooner than I found it again, generative. 00;33;10;19 - 00;33;44;13 AI So if you ask me when, we'll be ready for generally. AI Last year or two years ago, I would say not yet, but now we in the era where everything seems possible. So I remain extremely optimistic about generative in some of these last language models that will help us analyze unstructured data even more efficiently. Well, therein it's deeply fascinating and exciting stuff. 00;33;44;14 - 00;34;10;27 Thanks again for letting me pester you with these questions. If our listeners want to learn more about Sentinel, Operation Center or Mosaic or you, what's the best way for them to do that? So Sentinel has a poverty website where we post everything that we do. So is Sentinel initiative dot org. So I am a member of the Department of Population Medicine at Harvard Medical School. 00;34;10;29 - 00;35;00;16 So our website's population is a thought, but these would be two places that would be very informative for audience. Who wants to know more? All right. We appreciate that. And to our listeners, go ahead and subscribe to the show. Feel free to listen to past episodes because they are free. There's a lot to learn here. And if you want to learn more about how Oracle can accelerate your own life sciences research, just go to Oracle dot com slash life dash sciences and we'll see you next time on Research in Action.