KL Divergence
Linear Digressions - A podcast by Ben Jaffe and Katie Malone
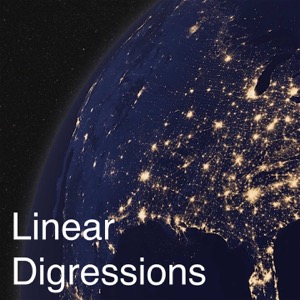
Categorie:
Kullback Leibler divergence, or KL divergence, is a measure of information loss when you try to approximate one distribution with another distribution. It comes to us originally from information theory, but today underpins other, more machine-learning-focused algorithms like t-SNE. And boy oh boy can it be tough to explain. But we're trying our hardest in this episode!