LM101-039: How to Solve Large Complex Constraint Satisfaction Problems (Monte Carlo Markov Chain and Markov Fields)[Rerun]
Learning Machines 101 - A podcast by Richard M. Golden, Ph.D., M.S.E.E., B.S.E.E.
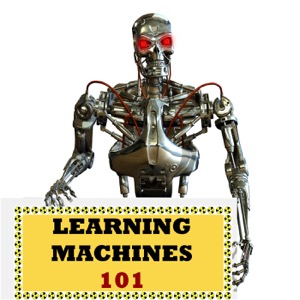
Categorie:
In this episode we discuss how to solve constraint satisfaction inference problems where knowledge is represented as a large unordered collection of complicated probabilistic constraints among a collection of variables. The goal of the inference process is to infer the most probable values of the unobservable variables given the observable variables. Concepts of Markov Random Fields and Monte Carlo Markov Chain methods are discussed. For additional details and technical notes, please visit the website: www.learningmachines101.com
Also feel free to visit us at twitter: @lm101talk