Meta-Learning for Robots – Intel on AI Season 3, Episode 12
Intel on AI - A podcast by Intel Corporation
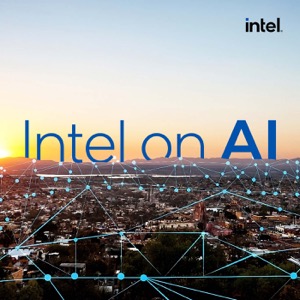
Categorie:
In this episode of Intel on AI host Amir Khosrowshahi and co-host Mariano Phielipp talk with Chelsea Finn about machine learning research focused on giving robots the capability to develop intelligent behavior. Chelsea is Assistant Professor in Computer Science and Electrical Engineering at Stanford University, whose Stanford IRIS (Intelligence through Robotic Interaction at Scale) lab is closely associated with the Stanford Artificial Intelligence Laboratory (SAIL). She received her Bachelor's degree in Electrical Engineering and Computer Science at MIT and her PhD in Computer Science at UC Berkeley, where she worked with Pieter Abbeel and Sergey Levine. In the podcast episode Chelsea explains the difference between supervised learning and reinforcement learning. She goes into detail about the different kinds of new reinforcement algorithms that can aid robots to learn more autonomously. Chelsea talks extensively about meta-learning—the concept of helping robots learn to learn—and her efforts to advance model-agnostic meta-learning (MAML). The episode closes with Chelsea and Mariano discussing the intersection of natural language processing and reinforcement learning. The three also talk about the future of robotics and artificial intelligence, including the complexity of setting up robotic reward functions for seemingly simple tasks. Academic research discussed in the podcast episode: Model-Agnostic Meta-Learning for Fast Adaptation of Deep Networks Meta-Learning with Memory-Augmented Neural Networks Matching Networks for One Shot Learning Learning to Learn with Gradients Bayesian Model-Agnostic Meta-Learning Meta-Learning with Implicit Gradients Meta-Learning Without Memorization Efficiently Identifying Task Groupings for Multi-Task Learning Three scenarios for continual learning Dota 2 with Large Scale Deep Reinforcement Learning ProtoTransformer: A Meta-Learning Approach to Providing Student Feedback