417: Hume AI with Alan Cowen
Giant Robots Smashing Into Other Giant Robots - A podcast by thoughtbot - Giovedì
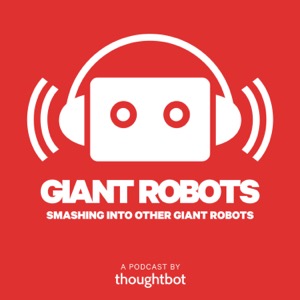
Dr. Alan Cowen is the Executive Director of The Hume Initiative, a non-profit dedicated to the responsible advancement of AI with empathy, and CEO of Hume AI, an AI research lab and empathetic AI company that is hoping to pave the way for AI that improves our emotional well-being. Chad talks with Alan about forming clear ethical guidelines around how this technology should be used because there is a problem in that the public is skeptical about whether technology is used for good or bad. The Hume Initiative is intended to lay out what concrete use cases will be and what use cases shouldn't be supported. Hume AI is built for developers to construct empathic abilities into their applications. The Hume Initiative Hume AI Follow Hume AI on Twitter or LinkedIn. Follow Alan on Twitter or LinkedIn. Follow thoughtbot on Twitter or LinkedIn. Become a Sponsor of Giant Robots! Transcript: CHAD: This is the Giant Robots Smashing Into Other Giant Robots Podcast, where we explore the design, development, and business of great products. I'm your host, Chad Pytel. And with me today is Dr. Alan Cowen, the Executive Director of The Hume Initiative, a non-profit dedicated to the responsible advancement of AI with empathy, and CEO of Hume AI, an AI research lab and empathetic AI company. Alan, thank you for joining me. DR. COWEN: Thanks for having me on. CHAD: That's a lot of words in that introduction. I'm glad I got through it in one take. Let's take a step back a little bit and talk about the two different things, The Hume Initiative and Hume AI. And what came first? DR. COWEN: So they were conceptualized at the same time. Practically speaking, Hume AI was started first only because it currently is the sole supporter of The Hume Initiative. But they were both conceptualized as this way to adjust two of the main problems that people have faced bringing empathic abilities to technology. Technology needs to have empathic abilities. If AI is going to get smart enough to make decisions on our behalf, it should understand whether those decisions are good or bad for our well-being. And a big part of that is understanding people's emotions because emotions are really what determine our well-being. The Hume Initiative addresses one of the challenges, which is the formation of clear ethical guidelines around how this technology should be used. And it's not because the companies pursuing this have bad intents; that's not the point at all. The problem is that the public is probably justifiably skeptical of whether this technology will be used for them or against them. And The Hume Initiative is intended as a way of laying out what the concrete use cases will be and what use cases shouldn't be supported. Hume AI is introducing solutions to the problem of how we build empathic AI algorithms. And the challenge there has been the data. So there have been a lot of attempts at building empathic AI or emotion AI, whatever you call it, basically ways of reading facial expression, emotion in the voice, and language. And there's been a few challenges, but most of them come down to the fact that the data tends to be based on outdated theories of emotion and/or it tends to be based on people's perceptual ratings of images largely from the internet or videos that are collected in more of an observational way without experimental control. And within those perceptual judgments, you see gender biases, sometimes racial biases, biases by what people are wearing, whether they're wearing sunglasses, for example, because people with sunglasses for some reason are perceived as being proud. [laughter] And the algorithms will always label people with sunglasses as being proud if you're training the algorithm that way. What you need basically is some way to control for people's identity, what they're wearing, get people's own self-reports as to what they're feeling or what they're expressing, and do it in a way that's somewhat randomized so that different people express a wide range of emotional behaviors in a wide range of contexts. And the contexts are somewhat randomized. So that's what we're doing with Hume AI is we're gathering that data, and it requires large-scale experiments to be run around the world. CHAD: In terms of the actual product that Hume AI is going to do, is it a standalone product? Or is it something that people building products will use? DR. COWEN: It's a developer product. It's built for developers to build empathic abilities into their applications. And so we are about to launch a developer portal, and we have a waitlist on our website on hume.ai for that. In the meantime, we've been licensing out the models that we're training and the data that we're using to train those models, which I actually kind of view as somewhat interchangeable. Models are basically descriptions of data. Some people have the resources to train those models on-premise; some people don't. But we're providing the solution to any developer who wants to build the ability to understand, for example, vocal expression into, say, a digital assistant. So can the digital assistant understand when you're frustrated and be able to change its response based on that information? And even potentially update the actual network, the neural network that's being used to generate that response, actually, backpropagate the fact that this was an unsatisfactory response and make the algorithm better. Is this something that you could use for health tech? So people are building out telehealth solutions that incorporate AI in various ways, one of which is can we get an objective classification of emotional behavior that can be used to help triage patients, send them to the right place, put them in touch with the right help? Can it be used to sub-diagnose disorders, diagnose disorders with more statistical power? Because you can incorporate more data and develop better treatments for those disorders, and that can be done in a wide range of contexts. CHAD: So you mentioned training AI models. I don't want to make the assumption that everyone knows what that means or looks like. Maybe if we could take a step back, if you don't mind, talk about what that maybe traditionally looks like and how Hume is actually different. DR. COWEN: Yeah, totally. When it comes to empathic AI, so this is an area where you're trying to train an algorithm to measure facial movements insofar as they have distinct meanings or measure inflections of the voice while people are speaking to understand the non-verbal indications of emotion in the voice. When you are training an algorithm to do that, you're taking in images, video, audio, and you're predicting people as attributions of emotion to themselves or to others and what people are feeling or what people say they're expressing or what other people say they hear in an expression. You need a lot of data for that. Traditionally, people have used smaller datasets and assumed that emotion can be reduced to a few categories. That's been one solution to this problem. And so basically, you'll have people pose facial expressions of anger, fear, happiness, sadness, disgust, and surprise, which are called the basic six emotions. And that was introduced by Paul Ekman in the 1970s. And there are whole datasets of people posing those six expressions or perhaps combinations of them. And usually, those facial expressions are front-lit and front-facing and meet certain constraints. And when you train a model on that data, it doesn't tend to generalize very well to naturalistic expressions that you encounter from day-to-day for a lot of different reasons; one is that the six basic emotions only capture about 30% of what people perceive in an expression. Another is that people in everyday situations have a wide range of lighting conditions, viewpoints, et cetera. And there's more diversity in age, gender than you see in these datasets and in ethnicity and so forth. And so, these algorithms don't generalize. Another approach is to get ratings of data from the internet. So there, you're not creating the dataset for this specific purpose. You're just scraping as many facial expressions or recordings of voices as you possibly can, maybe from YouTube. That's one way to scale up. That's one way to capture a much greater variety of naturalistic expressions. But then you're gathering ratings of these images. And those ratings are influenced not just by what somebody is expressing but also by somebody's gender, ethnicity, age, and what they're wearing, and so forth. CHAD: Well, in those scenarios, a person has also classified the image, to begin with, right? DR. COWEN: Yeah. CHAD: So someone is labeling that image as angry, for example. DR. COWEN: So typically, you're scraping a bunch of videos. You're giving them to raters typically from one country. And those raters are categorizing those images based on what they perceive to be the expression, and there are a lot of influences on that. If somebody is wearing a sporting outfit, and this is a hard bit of context to cut out, you can generally infer this person is likely to be expressing triumph or disappointments or all the different things people express when they're playing sports. And it's very different if somebody's wearing a suit. And so, these different biases seep into the algorithm. We did train probably the best version of this kind of algorithm when I was at Google. And we used it to study people's expressions in other videos from around the world, mostly home videos. And we found that people form expressions in characteristic contexts around the world. And the relationship between context and expression was largely preserved. We were looking at 16 facial expressions we were able to label accurately. And this was probably with the best version of an algorithm trained in this way. But we still only captured about half of the information people take away from expressions because we had to throw away a lot of the predictions due to these biases. So that's how algorithms are traditionally trained. Another way that you could go about it is by training a large model, like a large language model, if you're looking at an emotional language and query it in a special way. So let's say you take a GPT-3 kind of model, and you say, "Hey, what are the emotions associated with this sentence?" And there, you see exactly the same kind of biases as you'd see in perceptual ratings because typically, it's saying what is likely to be in that data. So it might say, "Well, pigeons are disgusting, and doves are beautiful," or something like that. And that's the kind of bias we don't care about. But you can imagine there are a lot of biases that we do care about in that data too. [laughs] And so, what's needed is experimental control. And I think this is actually when it comes to the things we really care about, something that people should consider more often in machine learning. What are the confounds that exist in the data that you're training an algorithm on? And if you really care about those confounds and you want to be scientific about it, about removing them, what's the solution? Well, the solution is to somehow randomize what somebody is expressing, for example. And that's what we do at Hume. We actually gather data with people reacting, for example, to very strongly evocative stimuli, which could be images, videos, paintings, et cetera, music. And we have balanced the set of stimuli in a way that makes it richly evocative of as many emotions as possible. And then what somebody is likely to be experiencing in a given setting is randomized relative to who they are since they see a random set of these stimuli or they undergo a random set of tasks. And so, to the extent possible, we've removed some of the relationships between ethnicity, gender, age, and what somebody is experiencing, or what they're expressing. And so we do this in a lot of different ways. And one thing you do is you can train on basically what is the stimulus that somebody was looking at instead of training on somebody's perception of an expression. CHAD: Hopefully, talking through this a little bit has helped people, one, I guess, understand why this is difficult. And that's where the need for a product by a company that specializes in it is important because it would be pretty difficult for a company just getting started to be able to do this in a scientifically controlled way. And in a sense, it's sort of like pooling the resources behind one product to do it well, and that can really do it well. You recently raised money pre-seed from investors. How obvious was the need to them, and how easy or hard was it for you to raise money? DR. COWEN: I had been basically in this world for a long time before I started Hume AI and The Hume Initiative. So during grad school, while I was publishing a lot of this science that was showing people's expressions were much more nuanced than a lot of these datasets and algorithms had considered before, I was getting inbounds from tech companies. And so, I worked a little bit with some startups. I worked with Facebook. I worked with Google. And I had seen this problem from a lot of different perspectives and viewpoints already. The need for data was very clear. The need for algorithms was clear because people literally had reached out to me and asked, "What are the best algorithms?" And I had to say, "Look, there are a few things, but all of them have problems. And they're mostly focused on the face, and you won't see much for the voice. And you won't see much for language." And what I had trained at Google was not something that was publicly available for facial expression. What is available for language probably the best one is another dataset that I helped put together at Google and algorithms trained on that called the GoEmotions dataset, which is used by Hugging Faces emotional language algorithm. And so I knew that there was this need, and a lot of people were looking for this kind of data, and so that's where it started. So talking to investors, it wasn't too hard to show them all the evidence that there was a need for this, a big market. And we raised a $5 million pre-seed. What we have spent a lot of that so far is in data collection. And that's made a huge difference in training algorithms for facial expression, voice, language, and so forth. And then what turns out to be more of a challenge is delivering those algorithms to people. And we're actually building a platform, an API platform, for that that will be really helpful in getting people started. CHAD: As you took on investors who, you know, they're trying to build a business. They want to create a business that gives them a return. And as you move towards a product in the marketplace, what are the things that you've encountered that are the biggest concerns in terms of the success? DR. COWEN: There's a scientific and sort of almost educational challenge. I think people have been fixated on a few ideas about emotion for a long time; these really sticky ideas like you can reduce emotion to six categories or two dimensions. So even when people take these really nuanced and accurate models that we've trained to distinguish 28 different kinds of facial expression, much broader array of facial expressions or 24 different kinds of vocal expression in vocal utterances like laughs, and cries, and screams, and sighs, and 16 different kinds of speech prosody, typically, people will take these, and they'll take out a few emotions, and they say, "Okay, well, this prediction is for the anger prediction, and that's the one I'm interested in." The challenge is in conceptualizing the phenomenon people are interested in classifying with these models and how they can relate that to what the model is predicting because typically, what constitutes anger is very different from one situation to another. Someone who's angry, who is maybe playing a sport, is going to be much more vocal about it than if you're on a customer service call. And that context is really important in going from an embedding that's general for different expressions, that can recognize 16 different emotional intonations in speech and fine-tuning it for that specific context. And I think that process can be difficult to understand if you're not fluent in the language of emotion science and particularly where it's gone over the last few years. And so part of what we're doing now is actually setting up ways to visualize the outputs of our models really smoothly and with any data so that people can navigate their data and actually see, okay, well, what this model is saying is an embedding of anger for what I'm interested in. Maybe it's customer service calls. Actually, it's a combination of a little bit of contempt and a little bit of disappointment in what people have labeled these expressions with. And now I can take this embedding, and I understand how to use it better. CHAD: Do you anticipate or hope for, and maybe those are the same, and maybe they're different, that you're going to have a few big customers or lots of small customers or something in between? DR. COWEN: We hope lots of small customers. [laughs] I want to get this into as many people's hands as possible. A lot of people are doing really innovative things in the startup world. There's also a huge need in big applications like digital assistants that are mostly in the hands of a few companies basically. We want to have an impact there as well. The difference, of course, will be the manner in which these solutions are delivered. The ease of providing people with APIs, subscribing to a pay-as-you-go model I think is really attractive for startups. And so that's how we're accessing that market. On the other hand, we do already have some big customers who are licensing the data or the models themselves. And I anticipate there will be a lot of that going forward as well. Mid-Roll Ad I wanted to tell you all about something I've been working on quietly for the past year or so, and that's AgencyU. AgencyU is a membership-based program where I work one-on-one with a small group of agency founders and leaders toward their business goals. We do one-on-one coaching sessions and also monthly group meetings. We start with goal setting, advice, and problem-solving based on my experiences over the last 18 years of running thoughtbot. As we progress as a group, we all get to know each other more. And many of the AgencyU members are now working on client projects together and even referring work to each other. Whether you're struggling to grow an agency, taking it to the next level and having growing pains, or a solo founder who just needs someone to talk to, in my 18 years of leading and growing thoughtbot, I've seen and learned from a lot of different situations, and I'd be happy to work with you. Learn more and sign up today at thoughtbot.com/agencyu. That's A-G-E-N-C-Y, the letter U. CHAD: You said you've built up to this point. But how long have you been working at it so far in terms of creating the actual product that will go to market soon? DR. COWEN: The company is only a year old. And we actually just had our first year anniversary. CHAD: Congratulations. DR. COWEN: [laughs] Thank you. Thank you. We are just now about to launch our platform, which I think is going to be our main product going forward. We're also running machine learning competitions in the research community, which there will be involvement from lots of tech companies and researchers around the world. So in many ways, we're still just getting started. But we have already what I think are the best models for understanding facial expression, the best models for understanding vocal utterances or what we call vocal bursts, which is actually different than understanding speech prosody or emotional intonation and language more generally. And we need separate models for that. We have both of probably the best models for those two modalities and are building what we think will be the best model for emotional language as well. And so we have solutions. Part of the product is delivering them, and that's what we're launching now. So we're at the beginning of that. CHAD: So has to get to this point taken longer than you were anticipating, the time you were anticipating or shorter? Did it go faster? DR. COWEN: I think my estimates for actually training these models and beating the state of the art were about on point. [chuckles] I mean, when we got started, I was ready to start running these experiments pretty quickly. So I designed all the experiments myself and started running them around the world, recruiting participants through labs, through consulting agencies, through crowdsourcing websites, a lot of different ways. There were a few challenges along the way, like figuring out how you could adjust the consent form in ways that weren't really relevant to ethics. And we had IRB approval. We had a very robust consent process for people to understand how their data was going to be used but were relevant to figuring out how you come up with language that is consistent with data privacy laws and each individual jurisdiction where you're running data collection. That took a little longer than I thought. [laughs] But suffice to say, we had the data. We had the models pretty quickly. I was able to recruit some of the top AI researchers in this space pretty quickly. We hit the ground running. We were able to take the data and train state-of-the-art models pretty fast. What's taking longer is getting the models into people's hands in two ways. I mean, negotiating enterprise contracts is always a struggle that many people are aware of. And then figuring out we needed to have a really user-friendly platform basically to deliver the models through APIs, and that's taking a little bit longer than anticipated. CHAD: So The Hume Initiative is a group of people that have come together and established some guidelines that companies sign on to in terms of what their solutions are going to take into account and do and not do. Do I have that right? DR. COWEN: Yeah. So we put together a separate non-profit. And we brought together some of the leading AI researchers, ethicists, with emotion scientists, and cyber law experts to this very unique composition of domain knowledge to develop what are really the first concrete ethical guidelines for empathic AI. Let's say for this use case; we support it if you meet these requirements. These are our recommendations. And for this use case, we don't support it. And we actually get really concrete. I think generally, with AI principles efforts or AI ethics efforts, people focus on the broad principles and left it to, I don't know, it's unclear often who is going to decide whether a use case is admissible or not under these principles. Because let's say they're codified into law, then it'll end up being a judge who doesn't necessarily have any knowledge of AI or emotion science or any of these things to say this is a use case that's consistent with these principles or not. We wanted to avoid that. And I think particularly; I think that the public is skeptical too of broader principles where they don't really know whether a given use of their data is compliant with those principles or not. I mean, sometimes it's easier. There are really good policies regarding surveillance that I think most of the big tech companies ascribe to where they say they won't be using your data in ways that you expect to have privacy and you actually don't. So I think there are pretty good principles there. There haven't really been good principles or concrete guidelines for what people might consider manipulative. And I think some technology that incorporates cues of emotion can be deemed manipulative in a sense. In the sense that you might not want to be sucked into a comment thread because something really provocative was shown to you after clicking on a notification that was unrelated to that. But the algorithm may have figured out this is a way to keep you in the app. [laughs] So that can be considered manipulative in some kind of way. I mean, it's bad if the person is vulnerable at that time. If the algorithm is able to read cues of your emotions and maybe through interoperability across different applications or because it knows it or has this information, this data already, it can say this is a person who is vulnerable right now to being provoked because they're in a bad mood. Maybe I can see that they just ordered food, and it's late, and it was canceled, whatever is. It can be any number of things. Or the way that this person queried a digital assistant or a search engine revealed this kind of emotional state. We don't want the algorithm to use that to get us to do something we otherwise wouldn't want to do So the principles we've set up around that are really important. Whenever somebody's emotional behaviors are involved or cues to their emotional state, they should be used to make sure that the algorithm is not using these cues against somebody or using them as a means to an end. What they should be used to do is make sure the algorithm is improving our emotions or improving our emotional state over time on average across many different people so that we're less frustrated on average over time, and we have more instances where we're satisfied, or content, or happy, or all inspired or whatever your measures or indicators of well-being you have present through these behaviors might be. The algorithm should be using these behaviors to enhance your well-being fundamentally. And wherever they're entering into an algorithm, we should be privy to how the algorithm is using them. And so that's essentially what the principles codify and make very concrete, and they say, "In this use case, this is how you can make sure this is the case, you know, health and wellness, digital assistants, photo-taking, arts and culture applications, film, animation." There are all these different applications of empathic AI. So it's a very broadly applicable thing because it applies to any text, any video with people in it, any audio where you hear people's voices. This is just a part of the data that's untapped relatively, or to the extent that it's tapped by algorithms today; it's done in a way that we don't really see, or maybe the developers don't even realize. If we make explicit that these are cues to people's emotions, there's a huge number of applications where we can then have algorithms learn from people's emotional cues and decide whether to enhance certain emotions or use them in certain ways. So I think it's going to be really, really key to get this right. And it requires expertise in how these emotions operate in daily life, in emotion science, in what is the definition of privacy here? What's the definition of a biometric measure which involves cyber law? And how does this intersect with existing laws and so forth? It's something that requires AI research expertise. You have to know how these algorithms work. It's something that requires specific kinds of AI ethics expertise. What is the alignment problem? How do we consider the value alignment in this situation? Which I think really comes down to optimizing for people's well-being. And we have brought together exactly that composition of expertise in The Hume Initiative. CHAD: Hume AI has sort of signed off and said, "We're going to follow these guidelines of The Hume Initiative." Does that apply to every customer who is a customer of Hume AI? DR. COWEN: Exactly, yeah. So we actually require people on our terms of use to adhere to the guidelines. And so, for a lot of people, that won't be that difficult because they'll look through the guidelines. They'll see that their use case is supported, that they're already following the recommendations that are in the guidelines. And so they're good. They're good to go. Some people might [laughs] see that they're not compliant with the recommendations. And then they'll be able to make adjustments to their product so that they're compliant. And then others who are pursuing use cases that are not supported by the ethics guidelines can't use the platform, which is exactly what we want. We don't want people using this for mass surveillance, for example, and that's stated pretty clearly in the guidelines. So yeah, we do require all of our customers to adhere to these guidelines that we've now launched at thehumeinitiative.org. CHAD: How important to you was it to have The Hume Initiative and these guidelines? Was it a precondition of doing all of this? DR. COWEN: Yeah, it was important for two reasons; one is that I felt that this shouldn't be used to exacerbate a lot of the problems that we're going to run into with AI eventually, if not already, where AI could be using our emotional behaviors to optimize for an objective that could be misaligned potentially with our desires, what emotions we want to feel, or with our well-being. Even though when you're privy to these emotional behaviors, you have the opportunity to do what a human does and say, "I have empathy. Therefore, I can say this is probably not a good way to get people to spend more time on this app or to buy this thing because I know that it's exploitative in some way." And I don't think that's the norm. I think, by and large, these strategies that have been used to optimize AI algorithms today have been good proxies for our well-being. Like, engagement is not necessarily a bad proxy for whether we want to spend time on doing something, but it's not good in all cases. And I think there's a huge amount of room for improvement because we don't know in all cases how the AI is getting us to be more engaged. And many of the strategies it uses may not be consistent with our well-being. But particularly going forward, once AI is smart enough, and once it has more control points in the environment, whether there are robots or digital assistants that have control over Internet of Things devices, AI will have an increasing influence on the environment around us, and it'll be smarter and smarter. And before long, it will be very important to make sure that it's aligned with our values. This is the concept of the alignment problem. Eventually, if you have a really, really smart, all-powerful, not all-powerful, but similar [laughter] powerful robot in your house and it's written by AI, and you tell it, "Hey, robot. I'm hungry. Make me the most delicious meal that you can that's healthy for me and satisfies all of these parameters using ingredients that are available in my kitchen." And if your cat happens to be in your kitchen and it realizes that, hey, this is lean meat. I have a great sense of what this person likes, so this is going to be really tasty. And it cooks your cat. [laughs] That's a way of satisfying this objective that you don't like. And so if it understood something about what is it that makes people happy by learning from our emotional behaviors in everyday life, we're not often saying to this robot, "This is something that I don't want you to cook." But if the robot understood this is something that makes you happy in everyday life, that would be one proxy for it to be able to figure out this would be a negative on your well-being if it did this. And so that is ultimately the solution. So we're going from; first, we at least want to optimize our algorithms existing today for people to feel better or indications of their well-being. And then, later on, we just want to make sure that, increasingly, that is the objective of these algorithms. I think that's been really important to me. CHAD: Obviously, it's not like the other companies out there doing this want to create a robot that cooks your cat. DR. COWEN: No. [laughs] CHAD: But it is possible that other companies don't prioritize it in the same way that Hume might. How do you stay motivated in the face of maybe not everyone caring about creating this in the same way that you are? DR. COWEN: That brings me to the other main reason for doing things this way, which is that I think there's enough of an economic incentive that you can create a company that is more successful for having made ethical commitments than otherwise. And I think that's particularly true if your company wasn't going to do anything unethical anyway, [laughs] which we didn't plan on doing and most companies don't plan on doing. Because if your company is not going to do anything unethical anyway, then you might as well be able to explain to people how you made the decision about what's ethical and what isn't and be able to make guarantees to them that actually attract more customers. Because the customers are able to say, "Look, they've made a legal commitment to not doing this." I don't have to suspect that these things are being used against me or in a manipulative way or in a way that doesn't preserve the privacy that I thought I had. I don't have to be skeptical of any of these things because I can see clearly that the company has made this potentially legal commitment, at least that's something that they're committed to publicly." So in that sense, it's purely an advantage. And that's true for AI generally but specifically for empathic AI. I think there's been a hunger for those kinds of ethical guidelines, and you can see it in how people react to news of this technology. There is generally a skepticism in the air. I think it goes back also to maybe sometimes people's concerns about privacy are legitimate if the question is whether what the output is picking up on is going to wind up in the hands of people you don't want it to end up in. And those people are privy to things about your lifestyle, or they're able to use that against you in some way. That is a real privacy issue. It's not necessarily, to me, a privacy issue. If an algorithm is processing these things on device and the data never goes anywhere, and it's only used in a way in which you actually want it to be used, which is maybe to surface better music to you or to have you be taking better pictures on your phone, these are all great things for you. And that data doesn't necessarily go anywhere in the same way that any of the photo data you take doesn't necessarily go anywhere even though it's already processed by lots and lots of algorithms, or your search queries aren't necessarily not private. Just because they're processed by algorithms, maybe even algorithms that are good for the business, they're not necessarily being seen by humans. And so, it's not necessarily a privacy issue. But people have this skepticism about emotion AI, in particular, empathic AI, in particular, because I think there are certain instincts that it plays on, like the idea that you're being watched. Early in our species history and even before our species, it was very important to be very wary of predators watching you from the bushes or from the crevices and all that. And I think that instinct is involved whenever we're being recorded, whenever there's a camera. And that's not just an issue for empathic AI but also for things like facial identification, which brings up legitimate privacy concerns but also, sometimes there are uses that we don't care about at all, or they're clearly good. Like, I think facial identification for unlocking your phone that's a really good use. And that is basically what it's used for by some companies. Some big tech companies are just using it for that and not much else. And so, when you unpack what you're doing with this stuff, it makes it a lot easier for people to be comfortable with it. And that is what the ethics initiative is doing essentially. It's giving people all of these use cases and recipes and unpacking what this is being used for so that people can be more comfortable with it. And I think that's actually something that is in the business' interest. CHAD: That's great. Well, I really appreciate, you know, there are a lot of pushes and pulls when founders are creating new companies. So to put a stake in the ground in terms of what's important to you and the right way to build this product and to go through the effort of creating these guidelines and a whole initiative around it and everything is...well, I can see that not everyone does that because of the concern around oh, is this going to hurt my business? Is it going to make it harder for me to succeed? And so when principals and business case align, great, but even when they don't, I think it's important, and I commend you for making sure that you're leading with your principals. DR. COWEN: Thanks. I mean, there have certainly been challenges to it. But I think that even so, the pros have outweighed the cons both ethically and for our business for us so far. CHAD: Great. So if folks have enjoyed today's conversation and either want to dig in more, you have a podcast, right? DR. COWEN: That's right. We have a podcast called The Feelings Lab, where we explore different emotions that are of concern in everyday life, that guide our everyday lives, and that are changing as a consequence of changes in society and technology. In Season One, we focused mostly on one emotion per episode. We had guests like Fred Armisen talking about horror, which is a really funny perspective to have [laughs] because fear is not always bad, and sometimes we like to watch horror movies. [laughs] And in Season Two, we're focusing particularly on the technology. And so we had the CEO of Embodied Paolo Pirjanian, who has a robot called Moxie that's used to help kids in their emotional development, and it's a great toy. We had the CEO, one of the co-founders of Soul Machines, which was an avatar company. We had VP at Omniverse Platform Developments in NVIDIA talking about how AI is changing the abilities of artists and changing basically the way that film is made. And it's very interesting. So I'd encourage people to check that out. CHAD: Where can people find that? I assume by searching for Feelings Lab in the podcast player. But do you have a domain name too? DR. COWEN: Yeah, you can go to hume.ai, and then you can go to our content hub. That's one way to find it. And you can find the podcast on Apple, SoundCloud, pretty much wherever you get podcasts. And we also have a YouTube channel, The Feelings Lab. Actually, I think the YouTube channel is Hume AI, and then we post content on The Feelings Lab there as well. CHAD: And you mentioned people can sign up now to be on the list for the Hume AI. DR. COWEN: So yes, if you are interested in building an empathic AI technology of any kind and you would like access to our voice models or face models, emotional language models, easy access, one-line API call for streaming or for files, pretty much any use case you might have, you can sign up for the waitlist at hume.ai. And we will be releasing a beta version of the platform over the next few months. CHAD: Cool. Well, if folks want to get in touch with you or follow along with you, where are the places where they can do that? DR. COWEN: Folks who want to get in touch, you can email [email protected] for information about our solutions, offerings, the company, or you can reach out to me personally [email protected] CHAD: Awesome. Alan, thank you so much for joining me. I really appreciate it. DR. COWEN: Thanks for having me. CHAD: You can subscribe to the show and find notes and transcripts for this episode at giantrobots.fm. If you have questions or comments, email us at [email protected]. You can find me on Twitter at @cpytel. This podcast is brought to you by thoughtbot and produced and edited by Mandy Moore. Thanks for listening, and see you next time. ANNOUNCER: This podcast was brought to you by thoughtbot. thoughtbot is your expert design and development partner. Let's make your product and team a success.Special Guest: Alan Cowen.Sponsored By:AgencyU: AgencyU is a membership-based program where Chad Pytel works one on one with a small group of Agency founders and leaders toward their business goals. You'll do one-on-one coaching sessions and also monthly group meetings. You'll start with goal setting, advice, and problem solving based on Chad's experiences over the last 18 years of running thoughtbot. As you progress as a group, you all get to know each other more and many of the AgencyU members are now working on client projects together and referring work to each other. Whether you’re struggling to grow your agency, taking it to the next level and having growing pains, or a solo founder who just needs someone to talk to, in his 18 years of leading and growing thoughtbot, Chad has seen and learned from a lot of different situations, and would be happy to work with you too. Learn more and sign up at thoughtbot.com/agencyuSupport Giant Robots Smashing Into Other Giant Robots