140 - Why Data Visualization Alone Doesn’t Fix UI/UX Design Problems in Analytical Data Products with T from Data Rocks NZ
Experiencing Data w/ Brian T. O’Neill (UX for AI Data Products, SAAS Analytics, Data Product Management) - A podcast by Brian T. O’Neill from Designing for Analytics - Martedì
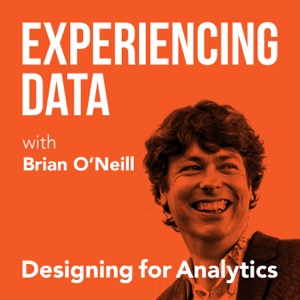
This week on Experiencing Data, I chat with a new kindred spirit! Recently, I connected with Thabata Romanowski—better known as "T from Data Rocks NZ"—to discuss her experience applying UX design principles to modern analytical data products and dashboards. T walks us through her experience working as a data analyst in the mining sector, sharing the journey of how these experiences laid the foundation for her transition to data visualization. Now, she specializes in transforming complex, industry-specific data sets into intuitive, user-friendly visual representations, and addresses the challenges faced by the analytics teams she supports through her design business. T and I tackle common misconceptions about design in the analytics field, discuss how we communicate and educate non-designers on applying UX design principles to their dashboard and application design work, and address the problem with "pretty charts." We also explore some of the core ideas in T's Design Manifesto, including principles like being purposeful, context-sensitive, collaborative, and humanistic—all aimed at increasing user adoption and business value by improving UX. Highlights/ Skip to: I welcome T from Data Rocks NZ onto the show (00:00) T's transition from mining to leading an information design and data visualization consultancy. (01:43) T discusses the critical role of clear communication in data design solutions. (03:39) We address the misconceptions around the role of design in data analytics. (06:54) T explains the importance of journey mapping in understanding users' needs. (15:25) We discuss the challenges of accurately capturing end-user needs. (19:00) T and I discuss the importance of talking directly to end-users when developing data products. (25:56) T shares her 'I like, I wish, I wonder' method for eliciting genuine user feedback. (33:03) T discusses her Data Design Manifesto for creating purposeful, context-aware, collaborative, and human-centered design principles in data. (36:37) We wrap up the conversation and share ways to connect with T. (40:49) Quotes from Today’s Episode "It's not so much that people…don't know what design is, it's more that they understand it differently from what it can actually do..." - T from Data Rocks NZ (06:59) "I think [misconception about design in technology] is rooted mainly in the fact that data has been very tied to IT teams, to technology teams, and they’re not always up to what design actually does.” - T from Data Rocks NZ (07:42) “If you strip design of function, it becomes art. So, it’s not art… it’s about being functional and being useful in helping people.” - T from Data Rocks NZ (09:06) "It’s not that people don’t know, really, that the word design exists, or that design applies to analytics and whatnot; it’s more that they have this misunderstanding that it’s about making things look a certain way, when in fact... It’s about function. It’s about helping people do stuff better." - T from Data Rocks NZ (09:19) “Journey Mapping means that you have to talk to people... Data is an inherently human thing. It is something that we create ourselves. So, it’s biased from the start. You can’t fully remove the human from the data" - T from Data Rocks NZ (15:36) “The biggest part of your data product success…happens outside of your technology and outside of your actual analysis. It’s defining who your audience is, what the context of this audience is, and to which purpose do they need that product. - T from Data Rocks NZ (19:08) “[In UX research], a tight, empowered product team needs regular exposure to end customers; there’s nothing that can replace that." - Brian O'Neill (25:58) “You have two sides [end-users and data team] that are frustrated with the same thing. The side who asked wasn’t really sure what to ask. And then the data team gets frustrated because the users don’t know what they want…Nobody really understood what the problem is. There’s a lot of assumptions happening t