#69 Getting Data Sharing Right at Netflix Scale - Interview w/ Justin Cunningham
Data Mesh Radio - A podcast by Data as a Product Podcast Network
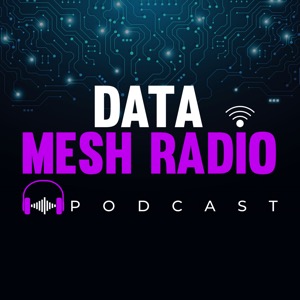
Categorie:
Sign up for Data Mesh Understanding's free roundtable and introduction programs here: https://landing.datameshunderstanding.com/Please Rate and Review us on your podcast app of choice!If you want to be a guest or give feedback (suggestions for topics, comments, etc.), please see hereEpisode list and links to all available episode transcripts here.Provided as a free resource by Data Mesh Understanding / Scott Hirleman. Get in touch with Scott on LinkedIn if you want to chat data mesh.Transcript for this episode (link) provided by Starburst. See their Data Mesh Summit recordings here and their great data mesh resource center hereJustin's LinkedIn: https://www.linkedin.com/in/justincinmd/In this episode, Scott interviewed Justin Cunningham, who worked as a tech lead and data architect on data platforms at Netflix, Yelp, and Atlassian over the last 8.5 years. In that time, Justin was involved in initiatives to push data ownership to developers / domains.To sum up one of Justin's points he touched on repeatedly - he recommends to create a pool of low effort data which will inherently have low quality. Use that for initial research into what might be useful. Focus on maximizing accessibility - you can have governance and use things like join restrictions or give consumers an ability to self-certify that they are using the data responsibly. Once you get the use cases, then you go for the data mesh quality data products. Justin saw a lot of success at Yelp focusing on data availability - getting data to a place it could be found and played with - was a bigger driver for success than focusing initially on data quality. Once people discovered what data was available and how they might use it, the organization was able to work towards getting that data to an acceptable quality level.Another point Justin made was figure out which you want to optimize for in general: getting things right upfront or testing and changing. He believes in optimizing for change. Create an adaptive process and optimize for learning. Keep it simple and focus on value delivery - it will set up more tractable bets.At Yelp, they were trying to ETL a huge amount of data in their data warehouse to build reports for the C-Suite. But they were never really going to get enough data ingested to really meet their goals. It was taking them 2 weeks to create each new set of ETLs and that was just creation, not maintenance - it was looking like they'd need 5x the number of people. What Justin found the most useful at Yelp was to focus on getting as much "usable" data in an automated way. They achieved this initially through the data mesh anti-pattern of copying direct from the underlying operational data stores and building business logic on top of it. But, that data getting into the hands of the data team meant there could be an initial value assessment - once they proved...