#289 Building the Right Foundations for Generative AI - Interview w/ May Xu
Data Mesh Radio - A podcast by Data as a Product Podcast Network
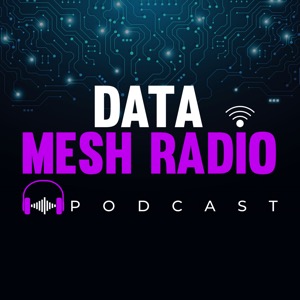
Categorie:
Please Rate and Review us on your podcast app of choice!Get involved with Data Mesh Understanding's free community roundtables and introductions: https://landing.datameshunderstanding.com/If you want to be a guest or give feedback (suggestions for topics, comments, etc.), please see hereEpisode list and links to all available episode transcripts here.Provided as a free resource by Data Mesh Understanding. Get in touch with Scott on LinkedIn.Transcript for this episode (link) provided by Starburst. You can download their Data Products for Dummies e-book (info-gated) here and their Data Mesh for Dummies e-book (info gated) here.May's LinkedIn: https://www.linkedin.com/in/may-xu-sydney/In this episode, Scott interviewed May Xu, Head of Technology, APAC Digital Engineering at Thoughtworks. To be clear, she was only representing her own views on the episode.We will use the terms GenAI and LLMs to mean Generative AI and Large-Language Models in this write-up rather than use the entire phrase each time :)Some key takeaways/thoughts from May's point of view:Garbage-in, garbage-out: if you don't have good quality data - across many dimensions - and "solid data architecture", you won't get good results from trying to leverage LLMs on your data. Or really on most of your data initiatives 😅There are 3 approaches to LLMs: train your own, start from pre-trained and tune them, or use existing pre-trained models. Many organizations should focus on the second.Relatedly, per a survey, most organizations understand they aren't capable of training their own LLMs from scratch at this point.It will likely take any organization around three months at least to train their own LLM from scratch. Parallel training and throwing money at the problem can only take you so far. And you need a LOT of high-quality data to train an LLM from scratch.There's a trend towards more people exploring and leveraging models that aren't so 'large', that have fewer parameters. They can often perform specific tasks better than general large parameter models.Similarly, there is a trend towards organizations exploring more domain-specific models instead of general purpose models like ChatGPT.?Controversial?: Machines have given humanity scalability through predictability and reliability. But GenAI inherently lacks predictability. You have to treat GenAI like working with a person and that means less inherent trust in their responses.Generative AI is definitely not the right approach to all problems. As always, you have to understand your tradeoffs. If you don’t feed your GenAI the right information, it will give you bad answers. It only knows what it